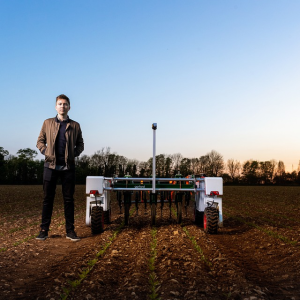
This paper outlines the systemic risks of using artificial intelligence (AI), specifically machine learning (ML) models, in agriculture and proposes ways of mitigating those risks. The three categories of risk considered are: risks related to data; risks related to the narrow optimisation of ML models; and risks linked to deploying ML models at scale.
In the first category, the authors note that multiple sources of data, such as agricultural research institutions, will not necessarily be usable at the same time because of different data formats or disorganisation. While there is much data on wheat, rice and corn, there is less on crops that are of the most importance to poorer or subsistence farmers, such as quinoa, cassava and sorghum. Meanwhile, there is limited data available on polyculture farming techniques, such as silvopasture, or other agricultural methods important in Indigenous food systems.
Risks related to narrow optimisation and unequal adoption of technology include: without deliberate effort to consider problems such as child labour and demographic discrimination, ML models will not factor these issues in and may therefore sustain systems that are problematic in this regard; small-scale farmers are likely to be excluded from the potential benefits of AI due to poor internet connectivity, marginalisation, and so on; and there are questions around the intellectual property rights of farmers, and the risk of smallholder farmers becoming dependent on proprietary systems.
The risks of deploying AI and ML at scale are argued to include: creating a few common points of failure, for example through the dependence of many ML models on common platforms such as TensorFlow and PyTorch; increasing the vulnerability of food supply chains to cyberattacks such as ransomware and denial-of-service attacks; and the potential for harmful recommendations of ML models, such as excessive fertilisation, being applied simultaneously over large areas of farmland, resulting in widespread crop failures or farms to ecosystems.
To address these concerns, the authors suggest that clear standards on data transparency and ownership rights should be followed. They suggest that the CGIAR’s Platform for Big Data in Agriculture (with which two co-authors are associated) provides tools to help gather data in line with good practices. They recommend “anticipatory” design of AI systems that would consider the ecological and social safety of potential ML recommendations. AI could be deployed in stages in what the authors call “digital sandboxes”, where potential failures can be tested and solved.
Abstract
Global agriculture is poised to benefit from the rapid advance and diffusion of artificial intelligence (AI) technologies. AI in agriculture could improve crop management and agricultural productivity through plant phenotyping, rapid diagnosis of plant disease, efficient application of agrochemicals and assistance for growers with location-relevant agronomic advice. However, the ramifications of machine learning (ML) models, expert systems and autonomous machines for farms, farmers and food security are poorly understood and under-appreciated. Here, we consider systemic risk factors of AI in agriculture. Namely, we review risks relating to interoperability, reliability and relevance of agricultural data, unintended socio-ecological consequences resulting from ML models optimised for yields, and safety and security concerns associated with deployment of ML platforms at scale. As a response, we suggest risk-mitigation measures, including inviting rural anthropologists and applied ecologists into the technology design process, applying frameworks for responsible and human-centred innovation, setting data cooperatives for improved data transparency and ownership rights, and initial deployment of agricultural AI in digital sandboxes.
Reference
Tzachor, A., Devare, M., King, B., Avin, S. and Ó hÉigeartaigh, S., 2022. Responsible artificial intelligence in agriculture requires systemic understanding of risks and externalities. Nature Machine Intelligence, 4(2), pp.104-109.
Read the full paper here. See also the TABLE explainer What is sustainable intensification?
Post a new comment »